I’ll start by saying that this is going to get a tad messy. This article…
The Categorisation Conundrum – Why Your Bank MUST Get This Right
[Warning: this is a super-nerdy post that might trigger some people. If you don’t like to geek out on Fintech data it might not be for you.]
I recently caught up with a chap called Jimmy Grafton at an establishment called the ‘Pub In the Park’, London Fields, E8, London. Jimmy, despite looking 12 years old, is something of a legend in the Australian Fintech community.
He lives there now, and I base myself there when I’m in London, which has been quite a bit recently. London Fields, that is. Not the Pub On The Park.
Although we both keep an office there. London Fields has been described as the “boujee part of Hackney” (by the Sunday Times, no less) and one of the best places to live in London. I highly recommend it.
Jimmy’s been up to his neck in Fintech since the halcyon days of 2019 (actually 2017-2019 was the official ‘Australian Fintech halcyon era’ but he was still in kindergarten then), before Fintech was too much of a thing and certainly before it became a party that people wanted to come to.
Back then the protagonists were building interesting & cool Fintechs, the likes of which we hadn’t seen before.
A 22 minute mortgage? No worries – thanks to Tiimely (formerly Tic:Toc Homeloans)
An app that buys shares with loose change when you shop? I got you – thanks to the OG, Raiz
An investment advisor in your pocket? Piece of p*ss, mate – cheers Stockspot (here’s my 2019 interview with Chris Brycki)
All your financial accounts showing easily on one screen? Meh, easy work – ta, Frollo
The hangers-on and observers were mostly true Fintech aficionados, dyed-in-the-wool power-users whose ideas and opinions actually informed the product roadmap of the start-ups they hung about with.
[Note: If you don’t have 100 Fintech apps on your phone (including a specific “graveyard of PFM” folder), you’re a noob in my eyes, and still on your trainer-wheels. And that’s totally fine.]
They were also, often, content creators, actually fuelling the ecosystem by putting out original content that was useful, relevant and insightful. Note the considered use of the word “creator” and not “curator.” Things in Fintech were somewhat cerebral before the great Covid dumbing-down. *Sigh*
Back then Jimmy put out a weekly Fintech newsletter called ‘The Inner Loop’ where he wrote about Fintech (funnily enough) in Australia (predominantly, but with a global lens, too).
There were product or system teardowns, new company information & gossip, and occasionally some other curated content drafted in from others (including a deep-dive I wrote on Australian PFM apps, here). But there was always something original & new to learn, especially in the deep-dives into payments or Open Banking or how Amazon works.
The writing was clean and elegant, and could’ve really been on any topic. Fintech just happened to be in his sniperish firing line.
And so, as we supped on our pints of Jupa, looking out over London Fields, lamenting the slow-decline of original Fintech content creation, we came up with a self-imposed challenge, a last hurrah, if you will.
Can you write an original, long-form blog post or article on the most mundane, prosaic topic and somehow manage to make it vaguely interesting and entertaining?
The winner shouts the Jupa at the next Pub On The Park reunion. Perhaps even a lunch at the 1 Michelin-starred Behind, conveniently located behind the POTP?
Perhaps not, in the current economic climate. A Pret-A-Manger sandwich and a pint, it is. Sad times.
Challenge accepted. Let’s see how I get on, and then see what Fintech’s very own Dorian Gray can come up with.
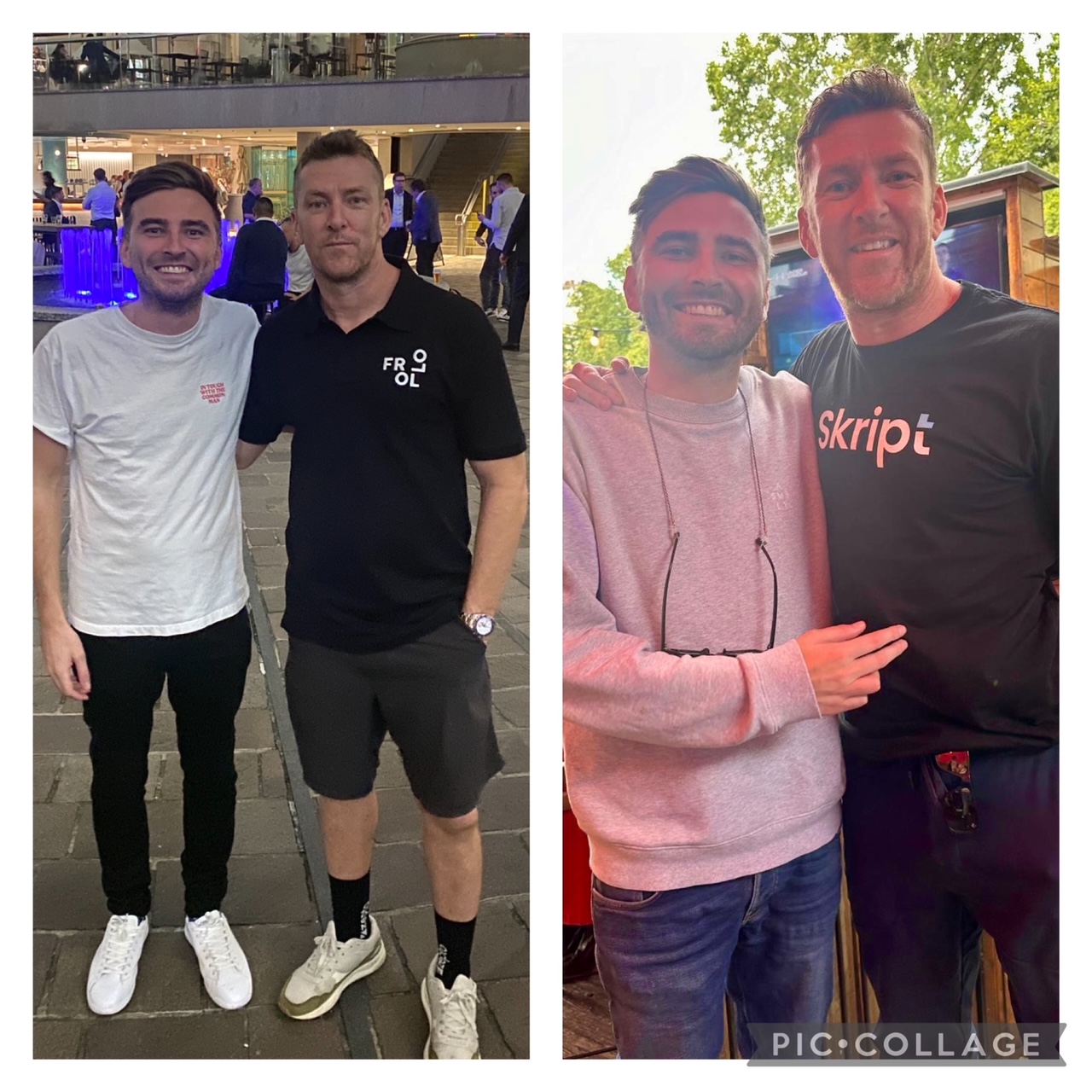
Introduction
In the last little while I’ve been asked by both a celebrated Fintech founder and a Fintech CEO for some pearls of wisdom to help them in their selection of a vendor for bank transaction categorisation / data enrichment.
This essay is a long-form summary of my responses.
I’m using the term “categorisation” and “data enrichment” interchangeably here. They are different, but part of the same process, and never seen without one another for too long, data’s Bunderberg & coke (or if you’re from England and old enough: Dempsey & Makepiece).
What is Financial Transaction Categorisation?
Well, I’d describe it to the layman thusly: it’s the process of analysing an individual’s or a business’ financial transactions in order to group them into specific groups or “categories” so that patterns of spending or money movement can be more easily identified, and useful insights about the spending can be provided.
Example:
Say I’m using a money management app (aka a Personal Financial Management (or PFM for short) app) to better manage my money, set some budgets and identify savings or better interest rates I could be earning.
The overall aim in using the app is to reduce my personal debt, or to work towards buying a house, or a car, or a baby (having a baby, not buying a baby. Although I think you probably can do that now.)
My bank account might have hundreds of transactions per month, all at random locations and with random vendors (or merchants), big and small.
If I have transactions peppered throughout a month at various fast-food restaurants, for example, it might be hard to identify these and hard to measure exactly how much I’m spending.
The categorisation process would group my Uber Eats, Door Dash, Chipotle, Mcdonalds spending together under the category of “fast-food restaurants” (for example) and I’ll be provided with the sad and astounding news that I spend $500 per month on junk-food.
This insight will (hopefully) be a wake-up call and I can try to modify my behaviour now that it is being measured.
The same can be applied to my spending on things like:
Transport – fuel, insurance, tyres, service
Entertainment – movies, pickleball court-hire, whale-watching cruise, err…OnlyFans
Household Goods – bedding, furniture, barbeque equipment
Loans – mortgage, car finance, Buy-now / Pay-Later
Through better understanding where my money is going, the better I can “Peter Drucker” my finances to achieve better financial outcomes. [Drucker, as we all know, said: “to manage anything, you must first measure it.”]
This is just the money management / budgeting use-case, too.
The same also applies when banks or other lenders are assessing your ability to pay back (or service) a loan.
It’s vital that they see where your money is going to better inform their credit models (i.e. whether and how much they’ll lend to you).
If a lender sees a whole bunch of Buy Now, Pay Later transactions grouped together in your financials, then they’re likely to deem you a higher-risk and not want a bar of you.
Why It’s Important?
Transaction details are the backbone of personal finance. They tell the story of where your money goes, what you prioritize, and how you manage your finances. With the rise of digital banking, people have become more engaged with their financial data.
Where Trouble Rolls Into Paradise – Key Pitfalls in Transaction Categorisation
There’s no avoiding it – categorisation / data enrichment is hard.
There are multitudinous factors that seem to have been brought into existence purely to fcuk with categorisation engines and algorithms.
And the days of good ole RegEX being able to fix things up are lonnnggggg gone.
Here are (just) some of the key challenges a categorisation engine comes up against.
1. When One Merchant Wears Many Hats
Yep, everyone should be able to identify that Coles or Woolworths fall into “groceries”.
But what if someone has been spending up at a Coles Express linked to a Shell petrol station (this is pre-sales of Coles Express so bear with me).
It’s “fuel”, right?
Well, yes. Perhaps.
But also, no.
If the spend at a Coles Express is over $100, say, then it’s reasonable to assume it’s a fuel transaction because, well, have you seen the price-gauging at Coles Express?
Items that usually cost $4 in a regular Coles have suddenly morphed into what, one can only assume, is the Faberge Egg equivalent, now costing $11 and, yet, looks exactly the same as the regular version.
But if the spend at Coles Express is less than $10, it’s hard to see how this could be fuel unless:
a) students are still putting $5 in the tank (note: I stopped doing this at least, what, 3 years ago).
b) someone is filling up my Vespa or a lawnmower. Who am I kidding? The Vespa costs $16 to fill up now.
But some lazy so-and-so’s would still not have rule-checked this and therefore mis-categorise it as fuel, thus throwing out the accuracy of the insights provided.
2. When Merchants Are Very Small Businesses (The Long Tail)
As discussed above, big well-known merchants (stores) should be easy enough for every categorisation provider to accurately identify – in Australia, think: Coles, Woolworths, JB Hi-Fi, Caltex (although also see above for how this can get tricky).
But what about the “long tail” of smaller merchants whose name isn’t well known or isn’t even synonymous with what they do?
Let’s use a couple of examples from around my local area:
- Misty’s
- Bob’s Place
- Bob’s Plaice
- Little L
- Vincent & Dupree
Unless you have previously categorised, observed, used offshore data workers these establishments, then you’re going to struggle to correctly identify them and the category they fall into and thus render any insights into spending habits or loan affordability less accurate than if you had matched them correctly.
For those interested, I’ve added the category for each below:
- Misty’s (Massage parlour / bordello)
- Bob’s Place (bar)
- Bob’s Plaice (fish & chip shop)
- Little L (chicken burger joint)
- Vincent & Dupree (hairdresser)
[Note: Bob’s Plaice doesn’t actually exist, but it would be interesting to know if a categorisation engine / model could pick up the variation in name. I’m somewhat doubtful.]
3. When Every Provider Mysteriously Has a 95% Accuracy Rate / Match Rate
A massive challenge for someone requiring categorisation services is that every supplier of categorisation services seems to have the exact same accuracy rate.
Who’d have thunk it?
Yep, dear reader, by some bizarre twist of data analytics fate, all purveyors of data enrichment (another fancy term for categorisation, basically) are able to identify & group bank transactions with a 95% accuracy level.
That is amazing and great for all of us.
We can all rest easy tonight knowing that our financial management insights or the accuracy of lending decisions being made about us are absolutely bang on the money, within a 5% error rate. That’s basically a rounding error.
The End.
Dang! We almost got away with it. We were within a gnat’s whisker of pulling it off.
But somebody caught it. Some Peter Falk in Columbo dude had to pause for dramatic effect just as he was half out the door, and say:
“there’s just one more thing. If all categorisation is at a 95% accuracy level , why have you categorised the Duke Of Gloucester (The DOG) Hotel which is a large and well-known pub in the Eastern suburbs of Sydney as “pet accommodation? And what does that say about the rest of your accuracy?”
And, while we’re on the subject, why is “Randwick Petroleum”, a petrol station, being identified as “miscellaneous” when it has the word ‘petrol’ in the title?
And that’s when, to quote the Travelling Wilbury’s in Tweeter & The Monkey Man:
…the walls came down,
All the way to hell,
Never saw them when they landed,
Never saw them when they fell.
But, let’s get back onto topic, instead of slip-sliding into a version of ‘Data Enrichment Hades’ that would make Dante’s Inferno seem like a casual marshmallow-toasting campfire.
Here’s why not every vendor is actually achieving the alleged industry-standard 95% accuracy rate, and how you can begin to pressure-test the claims.
a) There’s usually no easy way to test it.
Unless a categorisation provider has an app or direct-to-consumer product in-market then it’s really hard, nay impossible to accurately judge their categorisation accuracy.
If you send a sample file through to be tested, there’s a reasonable chance there might be a little bit of manual intervention along the way.
Personal Financial Management (PFM) apps like Frollo or Pocketbook, who build their own categorisation engines, were completely transparent in this regard because any potential buyer or user could simply download the app, link a bank account using screen-scraping or Open Banking and check the veracity of the transactions being categorised.
Interestingly, Pocketbook, which was the OG of PFM apps in Australia getting to 400k downloads and mobilizing an army of rapidly loyal fans, had fairly basic categorisation but allowed users to re-categorise incorrect transactions.
This shortfall turned into a, erm, longfall by creating extreme stickiness amongst users who, having taken the time to actively re-work their data, were now often on the hook to keep doing it to maintain some semblance of accuracy in the app.
But this is a bold strategy and, whilst it may have worked in 2016, might be harder to pull off now.
b) When “uncategorised” mysteriously becomes “general” expenditure = 95%
Most of the time, when the engine can’t identify the merchant or the category, it would be tagged as “uncategorised.”
Too many of these and it starts to look a bit like your categorisation engine doth butter no parsnips. Never a good sign.
So some cheeky scamps came up with a genius way of hiding their limitations.
Instead of admitting they didn’t have a clue who or what “ACME Products” was or is, and saying: “we can’t in all good conscience categorize this”, they simply tag all “uncategorised” transactions under “general” and suddenly your failure rate disappears.
You literally close the kimono and deny you have a problem.
“What’s your uncategorised rate?”
“Zero.”
“Oh wow. Impressive. Let’s work together.”
“Cool. I’ll get contracts sent over.”
Ah, Bosh! Deal done. Two ponies & a monkey, guvnor.
If only it were that simple.
Actually in many cases it is. Endless stories abound of businesses selecting a vendor without testing the efficacy of their enrichment. Caveat emptor has never been truer.
c) Is the 95% referring to the merchant identification accuracy or the category accuracy
It’s hard to explain this without getting completely into the weeds, but, in a nutshell, if someone is boasting of a 95% accuracy rate, it’s good to try and establish exactly what the 95% is referring to.
Is it “merchant” match rate?
Is it categorisation accuracy?
Is it the percentage chance that the moon landing actually happened?
Is it the likelihood that my Fintech t-shirt collection eclipses that of Rayn Ong? (in which case 95% seems high).
d) If you do test the data, there’s generally & without fail always an absolute howler ready to be the proverbial slippery banana skin
As I’ve alluded to, there’s always a rogue transaction lurking in the ether, waiting to try you up.
The ‘Dog Hotel’ is a pub, and not “pet accommodation” but the mistake is genuine and understandable. Which doesn’t stop it sticking out like a particularly sore thumb.
On one ”test” file I may or may not have, ahem, “heard” about, the results went back having been manually reviewed, just as a precaution. C’mon, it was a big deal with a potentially marquee prospect.
Our results file was reviewed by the prospect’s Chief Data Scientist who, whilst noting that the time taken to process the file indicated that there may have been a little manual jiggery-pokery, also commented on a “strange” transaction / classification that caught his eye, but he declined to highlight.
Upon poring over the file, we spotted it. A purchase of some “love beads” had somehow made its way into the “medical” category, whereupon it sat somewhat awkwardly, betwixt & between, never quite sure of its place in this world.
[And if that’s not life (as a financial data salesperson) imitating art, imitating life, I don’t know what is.]
But that was a banana skin, right there.
e) I did some analysis on my own transactions (an admittedly small sample size but one that i was on ‘better-than-cuddling’ terms with, and so should be able to measure fairly accurately) and found that nobody hit the mythical 95% benchmark (shock, horror!)
Every 3-4 months over a couple of years, I tracked the categorised of the same bank account transactions on 6 or 7 different Fintech apps who were using 5 or 6 different categorisation providers.
Was this a painful, laborious and inefficient process? Undoubtedly.
Did it help me understand some of the issues with transaction categorisation so I could better help the product team and, ultimately, the client understand what was going on? I’ve no idea but, hopefully, yes.
Here’s a snapshot from one round:
This shows a few things, but notably it illustrates just how varied categorisation results can be.
Take the “Transport for NSW” transactions of 4 & 5 February. Fairly straight-forward, you’d assume?
Three of six vendors correctly identified this bus ride as a ‘public transport’ transaction.
Two cheeky scampish vendors classified the transactions as “general” or “other.”
And one classified is as “sports,” presumably because the word “sport” is part of the word “transport.”
This reminds me of the time I was at a data consultancy and we had to screen mailouts going to “Scunthorpe” addresses. I’ll leave it to you to figure out why. [Scunthorpe is a town in the North of England.]
4. When Different Strokes Suit Different Folks
The other huge elephant at the categorization conference is that one man’s nectar is another man’s poison. Or one woman’s Primark is another woman’s Chanel. Or something.
The folks making decisions about who to use for categorisation a) may have had a particular experience with one provider that is at odds with somebody else’s. And there’s simply no legislating for this.
I thought I knew who was comfortably the best for lending categorisation, for example. [Lending categorisation differs from money management categorisation because it’s more concerned with things like discretionary & non-discretionary spend as opposed to deciphering between “bars & pubs” and “restaurants” as a money-management app might be.
And then a Fintech founder who previously worked for a lender told me that he had a team of folks spending days each month correcting the categorisation of the, so-called, best lending categorisation provider.
And that’s when I started to cry and question my belief systems.
Summary, Rankings And Recommendations
There’s no escaping it, transaction categorisation / data enrichment is a tough gig.
The pitfalls are many and the numerous slippery banana skins lurking mean that any slip-up is likely to be magnified.
That said, accuracy has improved markedly since I started working this patch back in 2017. Back then, things were largely based on Regular Expression word-matching, with often comedically atrocious results.
Unless you could pull off a Pocketbook, and have 400 “1,000 True Fans” to lift the Kevin Kelly essay title, you were left at the mercy of the incumbents or you had to start building your own categorisation engine, which is no small feat (although a bold few tried with varying results).
A.I (which I’ve deliberately avoided mentioning until, erm, word 2764) and machine-learning have helped enrichment models leap-and-bound forward in the past few years, nudging up accuracy of categorisation & merchant identification to previously unchartered success rates.
Data’s allure as the “new oil” may have waned somewhat of late, in the face of new shiny balls like NFTs (remember them) in 2021/22 and A.I in 2023, but, given the importance of lending in all of our financial lives, this is still incredibly fertile ground.
If I were brave enough or clever enough to be a Fintech founder for whom this really matters, and my data science team were telling me that we’re ‘top of the pops’ when it comes to categorisation accuracy, I’d get my own bank account transactions run through the engine and go through the results one-by-one – only then (and dependant on results, of course) would I have comfort that I’d solved the Categorisation Conundrum.
Over to you, Jimmy Grafton.
Oh, and for those still waiting for the rankings, I salute you. Your dogged persistence and ability to read this codswallop should be lauded. Here’s my top 5:
1. Looks Who’s Charging
Generally regarded as the gold standard for accurate categorisation, these guys stealthed into the market circa 2018 / 2019 and won contracts with multiple Big 4 banks. Never heard a bad word said about them. Sold to Experian.
2. UP Bank
I tried to sell data / categorisation to them in 2018 and they said they could build it better themselves. Bless, I thought, how cute. They were right, I was wrong. When you put their data into other people’s categorisation, it tends to break it because it shows how much better UP is.
3. Frollo
Build their own engine in maybe 2018/19 and have been continually refining it since, sometimes using my anaylsis. Not sure if this is good or bad, but improvements have been steady and ongoing.
4. Mogo Plus
A quiet achiever, used by two of the Big Four. Strong data science aproach.
In Fintech,
SFD